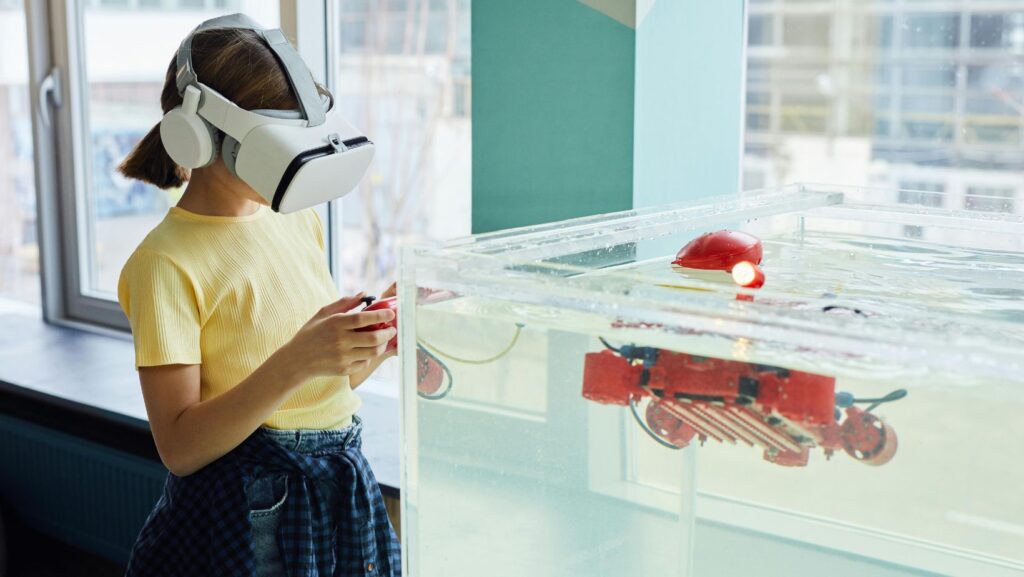
Probability is crucial in how artificial intelligence (AI) and machine learning systems make predictions, optimise algorithms, and analyse data. AI models rely on probability to handle uncertainty, helping them make accurate decisions even when information is incomplete. From speech recognition to self-driving cars, probability-driven approaches allow AI to function reliably in real-world scenarios.
Many AI applications depend on probability-based methods to improve performance. Recommendation systems use probability to suggest movies or products, while fraud detection models assess transaction risks based on statistical likelihoods. In healthcare, AI relies on probability to identify potential diseases and recommend treatments with the highest chance of success.
Probability in AI and Machine Learning
Artificial intelligence depends on probability to make sense of uncertain data. Instead of providing absolute answers, AI models work with probability scores to estimate the likelihood of different outcomes. This approach helps machines function in unpredictable environments where exact solutions are difficult to determine.
Many AI systems rely on probability distributions to assess patterns and trends. Speech recognition software, for instance, does not interpret spoken words as fixed values. Instead, it calculates probabilities for different interpretations and selects the highest likelihood. The same concept applies to image recognition, where AI assigns probability scores to identify objects within a picture.
A practical example of probability-driven AI can be found in gaming. Machine learning models can analyse betting patterns based on input from people who play real-time live roulette. Results from these models can help identify statistical trends that can influence the way bettors play.
While AI cannot predict outcomes with certainty, it uses probability to provide insights based on past data. These applications demonstrate how probability helps AI adapt and respond effectively to different scenarios.
Probability in Predictive Modelling
Machine learning algorithms use probability to forecast future events based on historical data. Instead of relying on fixed rules, AI examines past trends and determines the most likely outcomes. The more data it processes, the more refined its predictions become.
Weather forecasting provides a clear example of probability-driven AI. Meteorological models analyse past climate data to estimate the likelihood of rain, storms, or temperature shifts. Rather than making definitive statements, these models assign probability values to different weather conditions, allowing for more accurate forecasts.
In financial markets, probability helps AI-powered trading systems assess investment risks. Multiple variables influence market fluctuations, making it impossible to predict price movements completely. AI models calculate probabilities for stock price changes, enabling investors to make informed decisions. Industries that depend on forecasting, such as retail and supply chain management, also benefit from probability-based models to optimise operations.
Probability in Decision-Making Algorithms
Many AI-driven systems depend on probability to make complex decisions. AI evaluates risks and selects the most favourable option by assigning probability values to different choices. This method is commonly applied in robotics, automated systems, and real-time applications.
Self-driving cars are a prime example of probability-driven decision-making. Autonomous vehicles must assess multiple factors, such as traffic signals, pedestrian movement, and road conditions. Instead of making rigid decisions, AI assigns probability scores to potential hazards and adapts its responses accordingly. If a pedestrian is detected near a crossing, the car’s AI calculates the likelihood of movement and adjusts its speed to ensure safety.
Another AI technique, reinforcement learning, relies on probability to improve decision-making. AI systems in robotics and gaming environments learn through trial and error by assigning probability values to different actions. This allows AI to refine its strategies over time, leading to better performance in tasks such as robotic navigation and automated customer service.
Real-World Applications of Probability in AI
Many industries rely on AI-powered systems that use probability to enhance efficiency and accuracy. These applications help businesses make data-driven decisions while improving user experiences and security measures.
- Recommendation Systems: Streaming platforms, online retailers, and social media networks personalise user experiences through probability-based AI. Analysing browsing history and user interactions allows AI to predict content that aligns with individual preferences. Netflix, for example, estimates how likely a user is to enjoy a particular film based on past viewing behaviour.
- Fraud Detection: Banks and payment systems depend on probability-driven AI models to detect suspicious transactions. Fraud detection algorithms assess financial activities, assigning risk scores to identify unusual patterns. For instance, a sudden large withdrawal from an unfamiliar location may indicate fraudulent activity and prompt further investigation.
- Medical Diagnosis: AI supports healthcare professionals by predicting disease probabilities based on patient symptoms and medical history. Machine learning models compare thousands of cases to estimate the likelihood of specific conditions. This approach enhances early disease detection and helps doctors recommend effective treatments.
Probability enables AI to make informed predictions and smarter decisions in each field, benefiting businesses and consumers.
Conclusion
Probability is the foundation of AI’s ability to make predictions, optimise processes, and improve decision-making. Without it, machine learning models couldn’t handle uncertainty or adapt to real-world conditions. AI systems across industries—from fraud detection to self-driving cars—depend on probability to function accurately and efficiently.
Technology will continue evolving, and AI’s reliance on probability-based methods will grow stronger. Smarter, more adaptive AI models will emerge, refining how systems interpret data and make informed decisions. With probability shaping the future of AI, the possibilities for innovation are endless.